My colleagues and I recently published the paper, Theory-Guided Multiclass Text Classification in Online Academic Discussions, in the prestigious journal, Journal of Computer Information Systems. The paper presents on machine learning approaches to enhance our understanding of idea generation in asynchronous online discussions. Below is the tag cloud, abstract and full citation of the research.
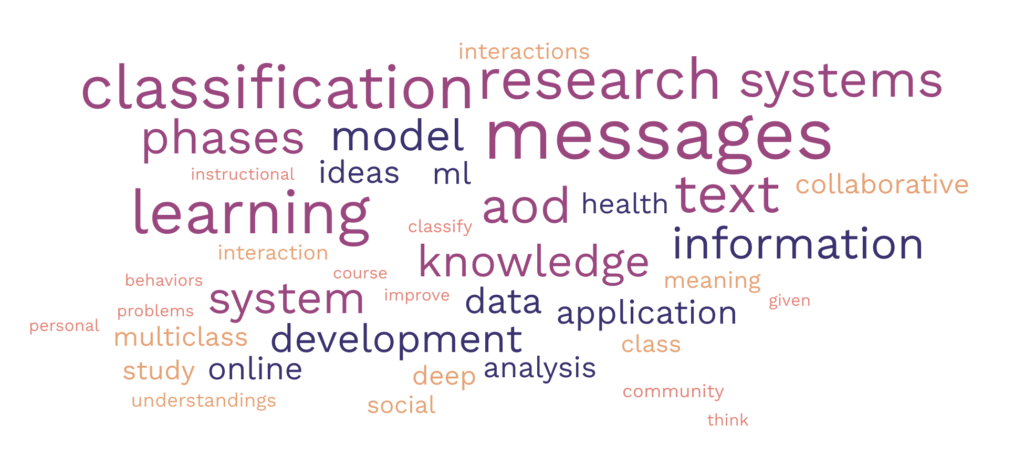
Abstract
Machine learning (ML) and deep learning (DL) provide significant opportunities to enhance our understanding of idea generation in asynchronous online discussions (AODs). Drawing on the interaction analysis model (IAM) as our theoretical framework, we built one baseline ML and three DL systems to automate message classification when assessing collaborative knowledge construction depth in academic AODs. The viability of these systems was demonstrated via four offerings of a traditional online course. We achieved 79% as the highest overall accuracy score across all phases of the IAM. To the best of our knowledge, this study is the first to classify AOD messages across all IAM phases. We contribute to the theory by updating the IAM to better explain how to promote deeper interactions in AODs. Additionally, we provide a methodological blueprint for future research where classifying text is crucial.
Reference
E. Eryilmaz, B. Thoms, Z. Ahmed. “Theory-Guided Multiclass Text Classification in Online Academic Discussions,” Journal of Computer Information Systems, 1–12, 2024. (Impact Factor 3.91)